top of page
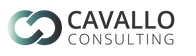
We are expert data strategy consultants.
Providing support to companies in adopting effective data management solutions for business application.
Cavallo Consulting & Partners S.r.l.
P.I. 13628901004
Viale Bruno Buozzi 102
00197 Rome
Italy
info[at]cavalloconsulting.it
bottom of page